Beyond the Base Case: Unlocking the Power of Scenario Analysis for Equity Research - Part I
How to identify critical factors, assess risks, and make informed decisions to better forecast earnings
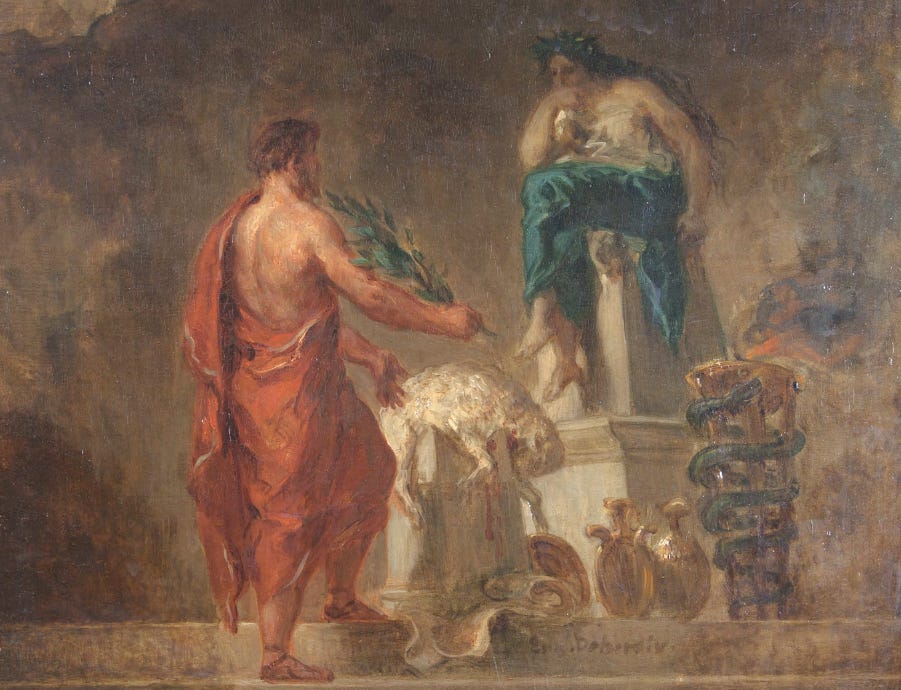
This is going to be a long piece, so I've provided a TL;DR of Part I below. My goal with this article is to explore all the possible implications and derivatives of how scenario planning can help analysts better forecast earnings and company performance. I hope you enjoy the topic as much as I did.
TL;DR
Scenario forecasting is a powerful tool for equity analysts to improve earnings estimates. It involves creating multiple potential futures based on different assumptions about key drivers.
Identifying and prioritizing critical factors is the key piece to effective scenario forecasting. These are the elements that have the most significant impact on a company's earnings. I share some interesting ways of uncovering critical factors.
The base case scenario is the most likely outcome, but upside and downside scenarios are important as well for understanding the range of possibilities. Assign probabilities to each scenario to reflect your assessment of their likelihood.
Real-time data and news monitoring are essential for adjusting forecasts and staying ahead of market events. Be prepared to adapt your scenarios as new information emerges.
Okay, let’s begin.
I was recently reading O'Connell’s Team America. In his book, he narrates how in the heart of World War II, General Dwight D. Eisenhower, the Supreme Allied Commander, faced a huge challenge: planning the D-Day invasion of Normandy.
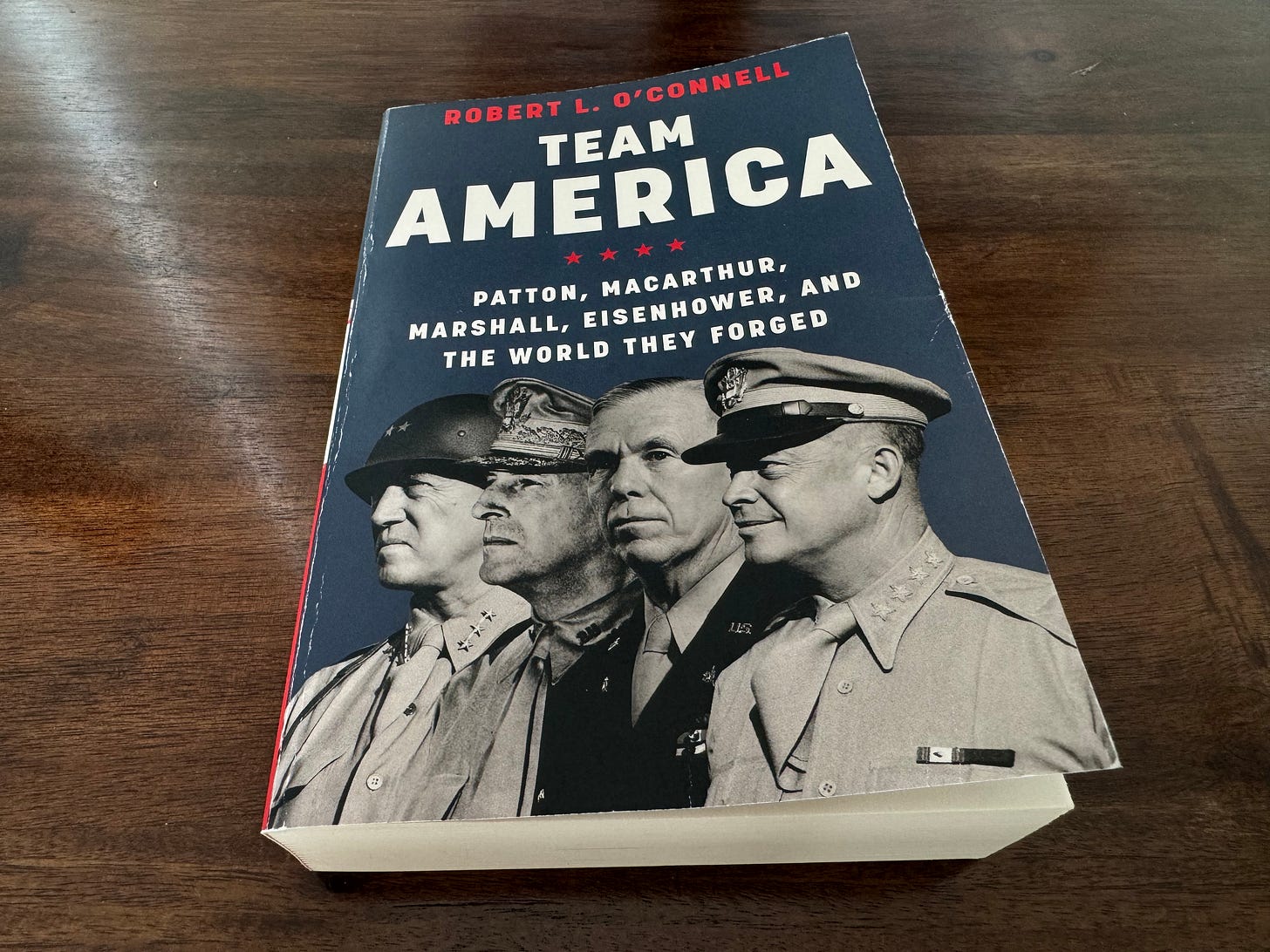
As you may know, the stakes were incredibly high, and the outcome of the war hung in the balance. Eisenhower knew that a single miscalculation could lead to catastrophic consequences.
To mitigate the risks and maximize the chances of success, Eisenhower and his team embraced a rigorous approach to scenario planning. They meticulously analyzed every aspect of the operation, from weather patterns and tides to enemy defences and troop movements. They developed multiple scenarios, each with its own set of assumptions and contingencies.
The base scenario envisioned a successful landing under favorable conditions, with Allied forces quickly establishing a beachhead and pushing inland. However, Eisenhower also considered a range of alternative scenarios, including adverse weather, fierce German resistance, and even a complete failure of the airborne assault.
So, what were the operation's critical success factors? According to O'Connell, Eisenhower was extremely worried about the moon and the weather, needing both for the operation to succeed.
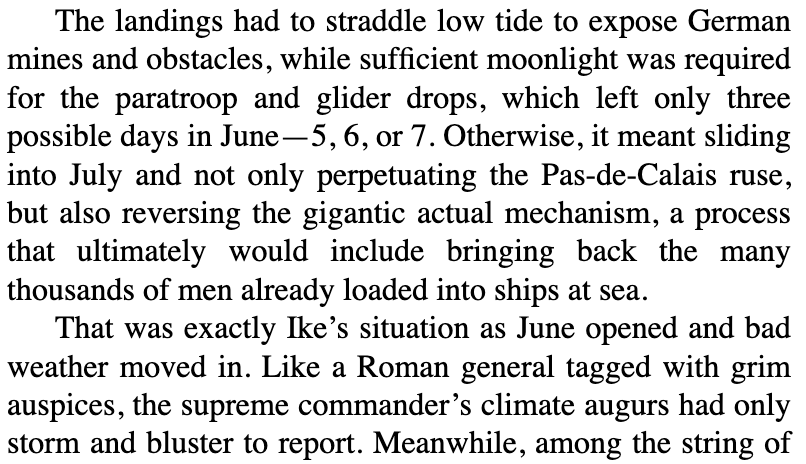
As a storm threatened to delay the whole invasion, on the evening of June 4 Eisenhower was weighing all possible scenarios.
Then, that same night, a weather aircraft far out in the Atlantic reported a high-pressure front moving quickly towards the east which would bring improved weather on June 5th, with clearing skies and calmer winds.
By nightfall of June 5th, conditions would be barely suitable for the airdrops and the sea landings the following morning, before worsening again later in the day. The window for the invasion would be a mere 24 hours.
With the fleet chief pressing to make a decision, in a meeting with his principal military commanders, and with this new set of probabilities for a favourable scenario, Eisenhower looked up and said to his commanders, to history, “Okay, we’ll go”.
D-Day invasion would ultimately liberate Western Europe, defeat Nazi Germany and end the Second World War.
Not to say that scenario analysis won WWII, but definitely became a staple in military planning.
In the corporate world, the stakes are not as high. But a framework based on scenario planning is an indispensable tool for equity analysts' forecasts.
I. Introduction
The Man Who Saw The Future
Pierre Wack was head of scenario planning at Royal Dutch/Shell Oil in London for more than ten years. He was a pioneer of scenario planning and practically the man who popularized the term in corporate culture.
Scenario planning helped Shell anticipate major events like the 1973 energy crisis and the fall of the Soviet Union, leading to outperform competitors.
But what were Wack's key insights?
He believed that the ability to see ahead was as important for managers as the ability to get things done.
He developed a process involving not just studying the business environment, but also examining one's own intent, competitive advantage, and strategic options.
Wack's methodology was never fully adopted at Shell. Nevertheless, he made a dent in corporate strategic thinking.
Now, how can we apply Wack’s teachings to equity analysis?
"Scenario planning, as Mr. Wack conducted it, provides precisely this kind of in-depth training over time. You research present key trends; you determine which are predictable and which are uncertain; you decide which uncertainties are most influential; you base some stories of the future on those uncertainties; you spend some time imaginatively playing out the implications of those stories; and then you use those implications to start all over again and develop a sense of the impending surprises that you cannot ignore.”
Kleiner, Art. "The Man Who Saw the Future." Strategy+Business, Booz & Company, 12 Feb. 2003.
From "If This, Then That" to Strategic Insight
Is this more than a glorified version of “if this, then that”?
Scenario forecasting is a method used to estimate a range of potential outcomes by considering various scenarios and their respective probabilities.
In the context of earnings estimation, this would be creating different projections for a company's future earnings based on varying assumptions about key factors that could impact its financial performance.
You would think about these factors, often called "critical factors," as macroeconomic conditions, industry trends, regulatory changes, and company-specific events.
By developing multiple scenarios, you can gain a deeper understanding of the potential risks and opportunities associated with an investment. This approach allows for a more comprehensive assessment of a company's earnings potential compared to relying solely on a single-point estimate.
II. Identifying and Prioritizing Critical Factors
At the heart of scenario forecasting is the concept of critical factors. These are the elements – internal or external to the company – that hold the power to significantly sway its earnings. I like to think of them as the levers that, when pulled, can send profits in one direction or another.
Why Critical Factors Matter
Magnifying effect: small changes in a critical factor can lead to disproportionately large impacts on earnings. For instance, a slight shift in interest rates might seem minor, but for a heavily indebted company, it could mean millions in extra interest expense, directly hitting the bottom line.
Domino effect: critical factors rarely act in isolation. A change in one can trigger a cascade, affecting other aspects of the business. A supply chain disruption, for example, might not only increase costs but also delay product launches, leading to lost sales and market share.
Risk and opportunity: Identifying critical factors is about more than just downside protection. It's also about spotting potential catalysts for growth. A breakthrough technology or a favorable regulatory change could be the wind beneath a company's wings, propelling its earnings to new heights.
How to Spot 'Em
Know the industry: every industry has its unique set of drivers. For tech companies, innovation and competition are paramount. For utilities, regulation and commodity prices are key. Thorough industry research is your starting point.
Deep dive into the company: what are the company's core strengths and weaknesses? Are they dependent on a single product or customer? What are their biggest cost drivers?
Look to the future: What emerging trends or technologies could disrupt the industry? Are there any upcoming regulatory changes that could impact the company's operations? Anticipating future developments is key to staying ahead of the curve.
Don't forget the macro: The broader economic environment plays a role. Interest rates, inflation, exchange rates, and even political events can have ripple effects on company earnings.
A Real World Example
One tool I like to use for quickly identifying critical factors in earnings reports is Koyfin's Analyst Estimates Trend. It clearly charts how EPS analyst expectations have evolved over time for upcoming calendar years.
Take Meta, for example.
There you can see that on February 1st, expectations for EPS over the coming four years changed dramatically. What happened that day? Sure enough, Meta held an earnings call where Zuckerberg provided us with the explanations. Meta outperformed earnings in Q4 2023 due to a combination of factors:
Strong revenue growth: Meta experienced a 25% year-over-year increase in total revenue, reaching $40.1 billion. This growth was primarily driven by a 24% increase in ad revenue, fueled by strong demand across various verticals, including online commerce, CPG, entertainment, media, and gaming. #critical factor number 1
Improved ad performance: Meta attributed its ad revenue growth to several factors, including increased ad impressions, higher average price per ad, and improvements in ad targeting and delivery through the use of AI and machine learning technologies. #critical factor number 2
Cost reduction: Meta implemented significant cost-cutting measures throughout 2023, which led to an 8% decrease in total expenses compared to the previous year. #critical factor number 3
Reels success: reels, Meta's short-form video format, continued to be a significant driver of engagement and revenue growth. The increased popularity of Reels and the company's ability to monetize it contributed to the overall positive financial results. #critical factor number 4
So, if I were trying to create scenarios to assess Meta's future earnings, I would look at:
The performance of the e-commerce, CPG, entertainment, media, and gaming verticals or, more generally, which is general state of the industry for Meta’s more important line’s of business.
Improvements in ad targeting technology.
News regarding cost-cutting or restructuring efforts, or plans for such measures.
Whether Meta has launched new ad formats or has plans to do so.
Prioritizing Critical Factors
Not all critical factors are created equal. Some are more likely to occur than others, and some have a more significant potential impact. To prioritize, consider:
Impact: how much could this factor affect earnings if it were to change? Use historical data, financial modelling, and expert opinions to estimate the potential magnitude. Look, if it’s earnings what we are trying to forecast we have to look at pricing, quantity sold and costs. Any factor that could affect some of those areas will have an impact on earnings.
Probability: how likely is this factor to change? This is where scenario analysis comes in handy. By considering different scenarios, I can assess the probability of various outcomes, but also have a good grasp on the news and press releases during the quarter to better assess this probability.
Now, as James Valentine1 puts it in his book, "...in a perfect world, you'd routinely uncover critical factors that: 1) have a large impact on the stock, 2) are likely to occur, and 3) are not in consensus. Unfortunately, many critical factors you come across will fall into only two of the three categories, which leaves you with little to offer..."
So, at this point I should probably have 3 or 4 critical factors that carry most of the weight and that I can realistically research. These are the factors that can substantially move the stock during my investing horizon.
"Realistically research" can mean different things to different people. Assuming you have 10 stocks in your portfolio and have identified 3 critical factors for each, you'd have to quantify 90 scenarios (3 scenarios per critical factor). This can quickly become a significant workload, meaning more work upfront but definitely helpful down the road.
III. Constructing Forecast Scenarios: Painting the Picture of the Future
Once I’ve identified your critical factors, it's time to build the scenarios that will guide your earnings forecasts. I like to think of these as different storylines, each with a distinct set of assumptions about how the critical factors will play out.
As a rule of thumb, and if I’m going to play with 3 scenarios, Valentine2 recommends using 50% probability for the base case, and 25% for the upside and downside scenarios.
The Base Case: The Most Likely Story (50% Probability)
This is my best estimate of how the company will perform under normal circumstances. It's not a pie-in-the-sky projection, but a realistic assessment based on current trends, historical data, and a moderate amount of optimism.
To create your base case:
Set reasonable assumptions: using historical averages, industry benchmarks, and expert opinions to determine reasonable values for the critical factors. For example, in a retailer, I might assume moderate economic growth, stable consumer spending, and manageable competition. You can always use Mauboussin’s Base Rate Book, more on this later.
Build the financial model: using the assumptions to project the company's income statement, balance sheet, and cash flow statement over my forecast period. This will provide me a baseline for the earnings estimate.
Stress test the model: don't just take the model's output at face value. Vary your assumptions slightly to see how sensitive your earnings estimate is to changes in the critical factors. This will help you gauge the level of uncertainty in your base case.
For The Base Case Always Use Reality Checks
And don't forget the trade-offs each company faces. If you're assuming price increases, will that affect market share? If you're assuming lots of growth, is that correlated with the required investments to achieve that growth (i.e., employee hiring and R&D)?3
For the base case, always use reality checks. See which are the pricing policies of the competitors, which are the historical growth rates of the industry, etc. The Base Rate Book is a great tool for reality checks while building your base case.
The Upside Case: The Best-Case Scenario (25% Probability)
This is the scenario where everything goes right for the company. The economy is booming, new products are flying off the shelves, and competitors are stumbling. It's important to note that this scenario should still be within the realm of possibility, not a fantastical dream.
To create your upside case:
Adjust key assumptions: identify the critical factors that could have the biggest positive impact on earnings and adjust their values accordingly. For example, you might assume higher revenue growth, improved margins, or a successful new product launch.
Re-run the financial model: with your new assumptions in place, re-run your financial model to see how much higher earnings could go under this optimistic scenario.
The Downside Case: The Worst-Case Scenario (25% Probability)
This is the nightmare scenario where everything goes wrong. The economy tanks, key customers defect, and a major lawsuit hits the company. Like the upside case, the downside scenario should be plausible, not a doomsday prediction.
To create your downside case:
Identify risks: what are the biggest risks facing the company? Could there be a major disruption in the supply chain? A shift in consumer preferences? A regulatory crackdown? Focus on the factors that could have the most significant negative impact.
Adjust assumptions: modify the values of your critical factors to reflect these risks. For example, I might assume lower revenue growth, increased costs, or a loss of market share.
Re-run the model: plug your new assumptions into your financial model to see how far earnings could fall under this pessimistic scenario.
A retailer example could look something like this:
Looking for Inflection Points
Most of the time the base case scenarios will fall really close to consensus thinking. So, how can you end up with scenarios that account for the Valentine’s Holy Trinity of critical factors? (those that (1) have a large impact on the stock, 2) are likely to occur, and 3) are not in consensus).
Those scenarios are likely to come up when there’s an inflection point in the future that has not been discounted by the market yet.
I’d say base case scenarios come up similar to consensus when extrapolating the current trend into the future. So if you know that some critical factor is going to have a huge change in the future, the could create some interesting scenario variations.
IV. Practical Tips for Earnings Estimation
Unearthing Insights That Matter
To create accurate and insightful scenarios, I need good quality of data. Suffice it to mention the old GIGO adage (garbage-in, garbage-out).
So, where can you find these golden nuggets of information that will help you grab hold of your critical factors? Well, I've mentioned some already, and I’m planning to write a good article on alternative sources of information, but now let’s stay with the following:
The usual suspects: start with the basics; company filings (10-Ks, 10-Qs, earnings transcripts), analyst reports, and industry research. But don't stop there. You could look beyond the obvious in trade publications, industry-specific databases, regulatory filings, and even social media for sentiment analysis.
Embrace the unconventional: consider alternative data sources. Think web scraping for competitor pricing, review sites, etc.. The insights you uncover here can give you a real edge.
Talk to the people: Interviews with industry experts, management teams, suppliers, and customers can offer invaluable insights not found in spreadsheets. These conversations can reveal a company's culture, challenges, and opportunities in ways that data alone cannot.
Turning Data into Actionable Intelligence
Once you've gather your data, it's time to sift through it and extract those golden nuggets of information that will drive your scenarios:
Spot the trends: look for patterns and trends in the data. Are certain metrics consistently outperforming or underperforming? Do specific events or announcements trigger predictable reactions? Use data visualization tools to help you spot these patterns.
Quantify the qualitative: whenever possible, translate qualitative insights into quantifiable metrics. For instance, if you hear that a company is planning a major expansion, try to estimate the potential impact on revenue and costs. This will help you integrate those insights into your financial models.
Balance the scales: always consider trade-offs when analyzing data. For example, if a company is raising prices, will it impact demand? If rapid growth is projected, are the necessary investments in place to support it? Understanding these trade-offs will lead to more realistic and nuanced scenarios.
Challenge your assumptions: don't take data at face value. Seek out opposing viewpoints and challenge your own assumptions.
Combining Qualitative and Quantitative Research
In the book Superforecasting, Phillip Tetlock mentions how a group of outsiders consistently outperforms the intelligence community.
If you read it you will find that there are several bits of useful information on how to make better forecasts, but two things caught my attention: how they constantly alternate between qualitative and quantitative information to improve their forecasts, and how they update their assessment every time there's a new piece of information.
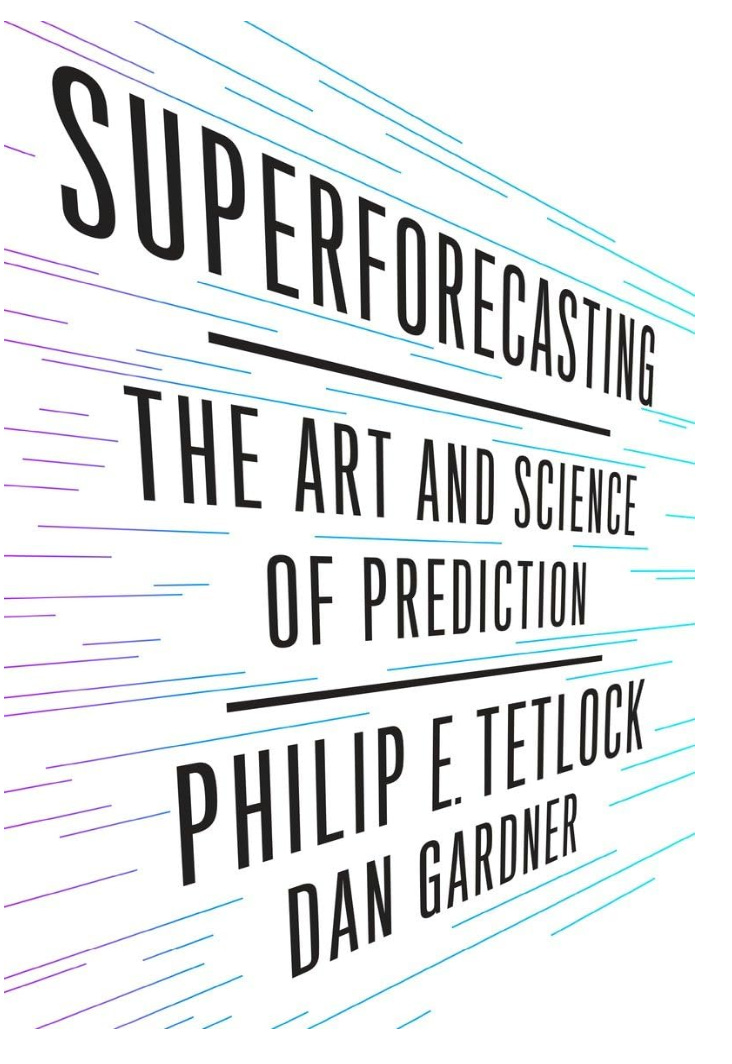
To truly excel at earnings estimation, I think it’s a bit of a mix between art and science. This means embracing both qualitative and quantitative research methods to create a more complete picture of a company's future.
Qualitative Research
I like to think that qualitative research is all about understanding the "why" behind the numbers.
Qualitative research can reveal important factors that may not be apparent in quantitative data. For example, a conversation with a company's CEO might uncover a strategic shift that's not yet reflected in financial statements. Or how he says things, his reactions to specific questions, body language, etc. That’s information that cannot be quantified.
Also, its useful to understand customer behaviour. Customer review websites or subreddits can shed light on customer preferences, brand perception, and buying patterns.
Quantitative Research
Quantitative research relies on hard data and statistical models to analyze trends, relationships, and probabilities. A few things here:
Test all assumptions: use quantitative models to test the validity of your qualitative assumptions. For example, if you believe a new product will be a hit, use market research data to estimate its potential market size and sales.
Be on the hunt for correlations: statistical analysis can help you identify correlations between different factors. For example, you might discover that a company's stock price is highly correlated with changes in commodity prices.
I like the saying “It is better to be vaguely right than exactly wrong”, so be mindful of the big picture while doing a deep dive into the hard data.
The Ever-Flowing Forecast: Adapting to Market Events with Agile Prediction
The world doesn't stand still, and neither should your forecasts. As I mentioned above, Tetlock argues that the best super forecasters are those who constantly update their forecasts based on new information.
A similar approach of constantly updating the business value of companies is shared by Turtle Creek in their "Tao of the Turtle."
Even a period with no news is a significant factor to update your probabilities. If you have a certain catalyst, the passage of time moving closer to that catalyst could make that event more probable.
Here's some best practices I can think of to keep forecasts updated:
Stay plugged in: monitor real-time news feeds, market data, and company announcements. Set alerts for keywords and topics relevant to your investments so you're not caught off guard by unexpected developments. I like to use Google Alerts, X alerts or even a tool called Visualping to notify me of changes in the press release’s page of different companies.
Assess the impact: when a significant event occurs, take a step back and assess the potential impact on critical factors. Will this event alter your assumptions about growth rates, margins, or competitive dynamics? Will this event change the forecasted earnings for your company?
Update your scenarios: if the event has the potential to significantly alter your forecasts, update your scenarios accordingly. Revise your assumptions, re-run your financial models, and adjust your probabilities.
Learn and adapt: every market event is a learning opportunity. Analyze what happened, why it happened, and how it affected your forecasts. Use this feedback to refine your models and improve your future predictions.
What I learned over time, is that scenario forecasting is not a one-and-done exercise. It's an ongoing process of learning, adapting, and refining your understanding of the forces that shape a company's financial performance.
This is the end of the first part of this article. In the second part I will discuss base rates ala Mauboussin, black swans and tail risks, asymmetric bets and psychological biases.
Sources
Valentine, J. J. (2011). Best Practices for Equity Research Analysts. McGraw-Hill Education.
Tetlock, P. E., & Gardner, D. (2015). Superforecasting: The Art and Science of Prediction. Crown Publishing Group.
Kahneman, D. (2011). Thinking, Fast and Slow. Farrar, Straus and Giroux.
Mauboussin, M. J., Callahan, D., & Majd, D. (2016). The Base Rate Book: Integrating the Past to Better Anticipate the Future. Credit Suisse.
Kleiner, A. (2003). The man who saw the future. Thought Leaders, Spring 2003(30). Booz & Company.
O'Connell, R. L. (2022). Team America: Patton, MacArthur, and Marshall, Eisenhower, and the World They Forged. Publisher.
Valentine, J. (2011). Best practices for equity research analysts: Essentials for buy-side and sell-side analysts (1st ed.). McGraw Hill.
Valentine, J. (2011). Best practices for equity research analysts: Essentials for buy-side and sell-side analysts (1st ed.). McGraw Hill.
Valentine, J. (2011). Best practices for equity research analysts: Essentials for buy-side and sell-side analysts (1st ed.). McGraw Hill.