Beyond the Base Case: Unlocking the Power of Scenario Analysis for Equity Research - Part II
Making investment decisions, black swans, and using base rates to anchor your forecasts
This is the second part of this article. If you missed the first one you access it here Beyond the Base Case: Unlocking the Power of Scenario Analysis for Equity Research - Part I.
As I began Part I of this series with a WWII event, the D-Day Invasion, it seems fitting to continue with the same theme. This time, let's shift our focus 8,400 miles away to the Pacific.
On a clear Sunday morning in December 1941, Admiral Husband E. Kimmel, Commander-in-Chief of the U.S. Pacific Fleet, stood on the deck of his flagship at Pearl Harbor. The harbor was calm, the mood relaxed. In Kimmel's mind, the threat of a Japanese attack on Hawaii was remote, despite rising tensions in the Pacific.
Kimmel's assessment wasn't baseless. U.S. military intelligence suggested that if Japan were to attack, it would likely be in the Philippines or Southeast Asia. The admiral's own analysis supported this view. After all, Hawaii was over 4,000 miles from Japan – surely too far for a surprise assault.
What Kimmel couldn't foresee was that he was about to experience a "black swan" event – an occurrence so rare and unexpected that it lay outside the realm of regular expectations. Well, maybe it wasn't so unexpected after all, but at the time, its probability was considered low for sure. Stay with me.
At 7:48 AM, the first wave of Japanese aircraft appeared over Pearl Harbor. By the time the attack ended two hours later, 18 U.S. ships were sunk or damaged, 347 aircraft were destroyed or damaged, and 2,403 Americans were dead.
Below, an image of the USS Pennsylvania; Kimmel's flagship.
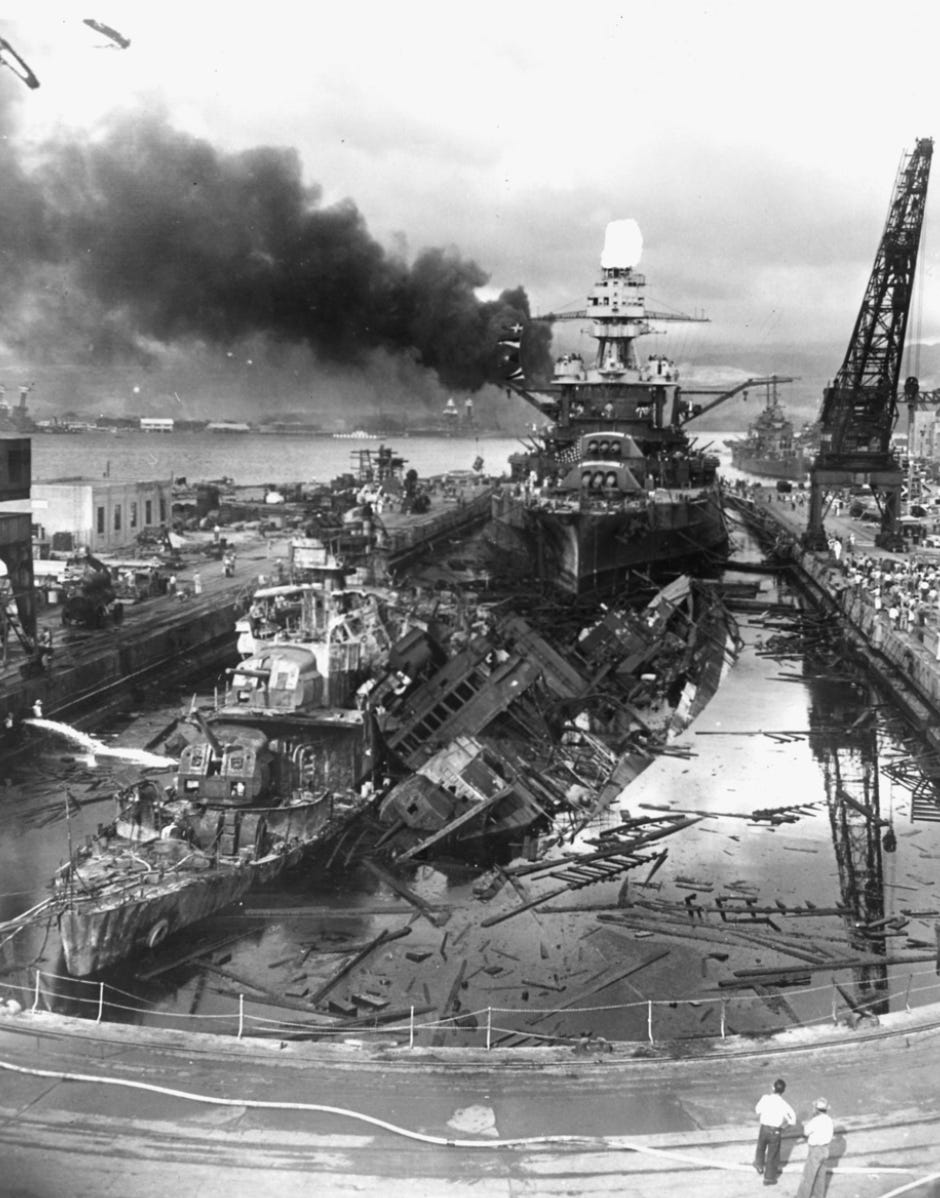
The Pearl Harbor attack serves as a stark illustration of how even experienced leaders can fall victim to psychological biases and fail to anticipate black swan events:
Confirmation bias: Kimmel and his staff interpreted intelligence in a way that confirmed their preexisting belief that Pearl Harbor was an unlikely target.
Normalcy bias: the idea that things will always function the way they normally have functioned led to a lack of preparedness for an unprecedented attack.
In the aftermath, Kimmel would reflect, "I never thought those little yellow sons of bitches could pull off such an attack, so far from Japan."1
So, as you continue reading, keep Admiral Kimmel's experience in mind. It reminds us that in an uncertain world, the improbable is not the same as the impossible. By understanding our biases and preparing for a wide range of scenarios, we can hope to navigate the choppy waters of investment with more success than the ill-fated fleet at Pearl Harbor.
Integrating Scenario Forecasting into Investment Decisions
Now, from Part I, we have our scenarios and we've considered the critical factors and their potential impact. The question is, how do we bridge the gap between these hypothetical scenarios and real-world investment decisions?
Scenario Planning for EPS Growth
As a best practice, we discussed that having 3 scenarios with roughly the following probabilities would be a useful starting point for scenario planning:
A base case with a 50% probability of happening.
A bull case with a 25% probability of happening.
A bear case with another 25% probability of happening.
Now, if I have different scenarios for EPS growth, what's important is to consider the full spectrum of outcomes, how wide the spread is between those scenarios, how good the bull scenario is, how bad the bear scenario is, and what the EPS growth expected for the base case is.
Not all scenario outcomes are created equal, and the result of each may well direct your buy, hold, or sell decision.
Assessing the Risk-Reward Profile and Deciding Wether to Buy/Sell/Hold
I'd say that everything comes down to the amount of dispersion you see in your EPS growth scenarios.
The first part would be to have a clear idea of what your threshold is for the bear case or losses. Have you assessed all the pertinent risks? If the bear case is a 2% decrease in EPS, and you estimate that that's the worst that can happen, then the upside would take care of itself.
I'd look at the downside first, and then, if the level of downside is acceptable or within my margin of possibilities, I'll look at the base case and the bull case.
As an investor, you have a certain and expected return for all investments, whether you compare against an index or if you have your own personal level.
The base case EPS expected growth should be above your threshold level. And then, if the bull case is really high, that's a cherry on top.
There are several assumptions I'm purposely omitting here. As investors, you care about total stock returns, and here we are talking only about EPS growth. Then, you would have your other estimates on an exit multiple.
Looking for Return Asymmetry
At the end of the day, you want to skew the odds in your favor as much as possible, and for that, you would be looking for asymmetries, having a small downside with a great upside, right?
So, looking for these asymmetries is easy once you have a lower level for your maximum downside and a base case level for your minimum required return.
In theory, everything looks really nice, but in practice, reality is messy, and there are many unknowns. At least by using this methodology, you would be able to quantify those known unknowns.
Now, what happens with the unknown unknowns? That's where the concept of the black swan by Nassim Nicholas Taleb comes in handy.
Black Swans and Tail Risk
Not all surprises are pleasant, right? Some come in the form of Black Swan events – rare, unpredictable occurrences that can send markets into chaos and greatly impact your company's earnings. Popularized by Nassim Taleb, these events remind us that the improbable can, in fact, happen.
Now, which are the key characteristics of black swans events? Let’s see.
Rarity: They lie outside the realm of regular expectations.
Extreme impact: Their effects are disproportionately large.
Retrospective predictability: They're often rationalized after the fact, making them seem predictable in hindsight, but of course by definition, you don’t expect them beforehand.
Black swans can impact company’s earnings in different ways:
You could see sudden revenue drops.
You can have unexpected costs or liabilities.
A supply chain disruption.
A rapid shift on consumer behaviour, etc.
Usually the concept of black swan is mentioned together with the concept of tail risks, which refers to the probability of extreme events occurring at the far ends – or "tails" – of a probability distribution.
The key difference is that black swans cannot be modeled or predicted using standard probability theory; they are unknown unknowns - events we couldn't have anticipated. Tail events, on the contrary, even with low probability, lie in the realm of the expected.
I know, I know, for the purists out there, Pearl Harbor would technically be categorized as a tail risk event. Nevertheless, the specific nature, timing, and scale of the attack were largely unforeseen, which aligns more closely with the characteristics of a black swan event.
We all know examples of black swans; COVID-19 Pandemic (2020), September 11 Attacks (2001), Fukushima Nuclear Disaster (2011), etc. These are event that had global impact and affected many industries at the same time.
Specifically talking about scenarios and earnings forecasting, how can we include a black swan analysis in the process? What tools or techniques can we incorporate? Let's take a look.
Identifying and Assessing Black Swans in Scenario Forecasting
I want to go beyond general market risks and dive deep into company-specific potential black swan events. Below, I share several approaches to help you identify and assess these type of events for a specific company.
Reverse Stress Testing
Similar to pre-mortem analysis, reverse stress testing starts with the assumption that the company has failed or experienced a catastrophic loss, to then work backwards to identify what could cause such an outcome.
Process:
Define a "failure" scenario (e.g., 50% drop in revenue, bankruptcy)
Brainstorm potential causes that could lead to this outcome
Assess the plausibility of each cause
Example: For a semiconductor company, a "failure" might be losing 70% market share. Working backwards, you might identify risks like:
Breakthrough technology by a competitor
Critical patent invalidation
Massive quality control failure leading to recalls, etc.
Supply Chain Deep Dive
Analyze the entire supply chain to identify critical dependencies and potential points of failure.
Process:
Map out the complete supply chain
Identify single points of failure or critical bottlenecks
Consider geopolitical risks for each key supplier location
Assess natural disaster risks for crucial manufacturing sites
Example: For an electric vehicle manufacturer, you might discover:
80% of a critical battery component comes from a single supplier in a politically unstable region
The only factory producing a specialized chip is in an area prone to earthquakes. In general, I’m looking for resilient companies, this was an important point analyzing the home appliances manufacturer Shark Ninja where supplies play an outsize rol in company performance.
Regulatory Scenario Planning
Imagine extreme regulatory changes that could significantly impact the company's business model.
Process:
Identify key regulations affecting the company
Envision worst-case regulatory scenarios
Consider potential new regulations that could disrupt the industry
Example: For a social media company, regulatory black swans might include:
Mandatory user age verification laws
Extreme data privacy regulations requiring deletion of all historical user data
Classification of the platform as a publisher, making it liable for user content
Technology Disruption Analysis
Consider how emerging or hypothetical technologies could render the company's products or services obsolete. Yes, I know, it's really difficult to do, but if you have something a ChatGPT launch for example, try to assess the probability of future iterations affecting your business (look at what happened to Chegg):
Process:
Research cutting-edge technologies in the industry
Imagine potential technological leaps, even if they seem far-fetched
Assess how these technologies could impact the company's value proposition
Example: For a traditional bank, technology black swans might include:
Quantum computing making current encryption methods obsolete
May be you could have AI systems capable of providing more accurate credit assessments than traditional methods
Historical Analogy Method
Study historical black swan events in similar industries or companies, then consider how analogous events could affect the company you're analyzing, or look at competitors with more history and see which black swans they endured in the past.
Process:
Research past black swan events in the industry
Identify similar companies that have faced unexpected crises
Draw parallels to your target company
Imagine how similar events could unfold in the current context
Example: When analyzing an oil company, you might look at:
The BP Deepwater Horizon oil spill
The 1973 oil crisis
The rapid rise of shale oil production
Then, consider modern analogues like:
A major spill from deep-sea mining operations
A sudden, coordinated global ban on new fossil fuel extraction
A breakthrough in fusion energy technology
Science Fiction Scenario Planning
Use speculative fiction techniques to imagine highly unlikely but potentially impactful future scenarios.
Process:
Start with current trends and extrapolate them to extreme conclusions
Imagine how these scenarios would impact the company
Work backwards to identify early warning signs or triggers
Example: For a luxury car manufacturer, sci-fi scenarios might include:
Extreme climate change events world wide that end up in regulations banning private car ownership
Neural interfaces allowing direct mind control of vehicles, changing the entire driving experience, I guess you get the idea…
Remember, the goal isn't to predict these events accurately, but to expand the scenario planning process to include a broader range of possibilities, improving your overall risk assessment and decision-making process.
The Importance of Base Rates (à la Mauboussin)
Imagine you find an amazing company. They have innovative products, they operate in an expanding industry with a lot of tailwinds, and they have an outstanding CEO. You are thinking, "This stock is a winner."
Now, how often do companies like this actually achieve their optimistic goals? This is where base rates and Mauboussin’s Base Rate Book come in.
Why Base Rates Matter in Forecasting?
In essence, base rates are historical odds. They tell you how frequently a particular event or outcome has happened in the past under similar circumstances for a specific class. For example, the base rate for a new drug gaining FDA approval might be 10%, meaning 9 out of 10 drugs fail to make it to market.
Base rates act as a reality check, grounding your forecasts in historical data and preventing you from getting carried away by the hype or your own optimism. I like to think of them as the voice of experience, whispering, "Hold on a minute, how often does this really happen?"
Michael Mauboussin has also advocated for the use of base rates in his book "Think Twice". He argues that ignoring base rates is common for investors, leading to overly optimistic forecasts and disappointing results.
You just have to take a look at the graph comparing the 3-Year Net Income CAGR estimates vs. the actual occurrences:
You can see that the estimates are much more concentrated than the actual compounded annual growth rate base case.
Integrating Base Rates into Scenario Forecasting
Now that we understand the importance of base rates in tempering our expectations, let's explore how we can practically incorporate them into our scenario forecasting process.
Identify relevant base rates: start by asking, "What's the historical track record for companies in this industry, with this business model, or facing similar challenges?" Mauboussin uses revenue size. Look for data on average growth rates, profit margins, market share changes, and the frequency of specific events like mergers or bankruptcies.
Refine your probabilities: once you have the base rates, use them to adjust the probabilities you assigned to your scenarios. If your base case is far rosier than historical averages, it might be time to dial back. Conversely, if your downside scenario seems overly pessimistic, the base rates might suggest giving it more weight.
Blend with specific knowledge: base rates are not a crystal ball. They provide valuable context, but they don't account for the unique nuances of each company or situation. Combine base rate data with your company-specific research, expert opinions, and qualitative insights to arrive at a more holistic and accurate forecast.
Basically, start with the base rate and then adjust based on your specific knowledge of the company.
Limitations of Base Rates
Yes, there are some limitations:
The past doesn’t always repeat itself: what worked in the past might not be a reliable predictor of future performance.
Outliers exist: exceptional companies or disruptive innovations can defy base rates and achieve extraordinary results, just look at NVDA. Relying solely on historical averages might blind you to these opportunities.
Sample size matters: if the historical data set is small or biased, the base rate might not be statistically reliable.
Relevance is key: historical data may not always be relevant to current market conditions. Economic cycles, geopolitical shifts, and technological advancements can all render past trends obsolete.
Therefore, you need to view base rates as one piece of the process, not the entire picture. Combine them with other forecasting techniques to create a more comprehensive and nuanced understanding of the investment landscape.
Let’s now transition to another important aspect of forecasting, being mindful of psychological biases.
Psychological Biases and Their Impact on Forecasting
The topic of psychological biases is really extensive, and I plan to do a whole article or series of articles referring to them. Nevertheless, I think it's worth mentioning the ones that are more prone to appear while creating earnings scenarios.
Psychological biases can significantly skew our judgment, leading to inaccurate forecasts and suboptimal investment decisions. So, below, I'm sharing the most common biases and some strategies to combat them.
Common Cognitive Biases Affecting Analysts' Judgment
Overconfidence Bias
Description: the tendency to overestimate one's own abilities or the accuracy of one's forecasts. You know… that famous Svenson2 study where 93% of U.S. drivers rated themselves as better than the median in terms of driving skill.
Impact on forecasting: can lead to overly narrow range of scenarios or underestimation of potential risks.
Anchoring Bias
Description: the tendency to rely too heavily on the first piece of information encountered when making decisions.
Impact on forecasting: can cause analysts to insufficiently adjust their forecasts based on new information. This could be for example fixating on a company's previous year's earnings when projecting future performance, despite significant changes in the business environment.
Confirmation Bias
Description: the tendency to search for, interpret, and recall information in a way that confirms one's preexisting beliefs.
Impact on forecasting: can lead to overlooking contradictory evidence and reinforcing incorrect assumptions.
Example: an investor bullish on a stock might focus on positive news while downplaying negative indicators. That's why it's important to always argue both in favor of and against a position.
Recency Bias
Description: the tendency to place greater importance on recent events compared to those in the more distant past.
Impact on forecasting: can result in overreacting to short-term trends and underestimating long-term patterns.
Availability Bias
Description: the tendency to overestimate the likelihood of events with greater "availability" in memory.
Impact on forecasting: can lead to overemphasis on dramatic or recent events in scenario planning.
Example: overestimating the probability of a market crash due to vivid memories of the 2008 financial crisis.
Groupthink
Description: the practice of thinking or making decisions as a group, resulting in unchallenged, poor-quality decision-making.
Impact on forecasting: can lead to consensus forecasts that fail to consider a wide range of possibilities.
Example: a team of analysts all agreeing on a bullish outlook without critically examining potential downsides.
Strategies for Mitigating Biases and Improving Objectivity
So, what do you do if you want to avoid these psychological biases? Over the years, I have come across different techniques and approaches that have helped me think more clearly. Some I have already mentioned in part one of this article. Please find a complete list below.
Utilize Pre-Mortem Analysis
Strategy: imagine the forecast has failed and work backwards to determine potential causes.
Benefit: helps identify overlooked risks and challenges assumptions.
Seek Diverse Perspectives
Strategy: actively seek out viewpoints that contradict your own, both from team members and external sources.
Benefit: counteracts confirmation bias and groupthink.
Utilize Checklists and Structured Processes
Strategy: develop and use standardized checklists for scenario development and forecast reviews.
Benefit: helps consistent consideration of key factors, reducing the impact of cognitive shortcuts.
Conduct Post-Forecast Reviews
Strategy: regularly review past forecasts, analyzing both successes and failures. You need to create feedback loops to learn what went right and what went wrong.
Benefit: improves calibration and helps identify persistent biases.
Practice Mindfulness and Metacognition
Strategy: cultivate awareness of your own thought processes and emotional states during analysis. I’m planning to write much more about this in the future, if you are particularly interested in some specific topic you can comment below.
Benefit: helps recognize when biases might be influencing your judgment.
The goal is not to eliminate biases entirely (which is probably impossible) but to recognize their influence and implement strategies to counteract their negative impacts on decision-making.
Conclusion: Embracing Scenario Forecasting for Better Earnings Estimation
Hopefully, you now have a more complete vision on how to use scenario forecasting as a tool to significantly enhance the accuracy and reliability of earnings estimates.
By incorporating key concepts such as base rates, black swans, asymmetric bets, and an awareness of psychological biases, analysts and investors in general, can develop a more comprehensive and nuanced understanding of potential future outcomes.
Key Takeaways
The power of base rates: anchoring forecasts in historical data provides a solid foundation for analysis. Base rates offer a reality check against overly optimistic or pessimistic projections, helping to calibrate expectations within realistic bounds.
Preparing for black swans: while rare and unpredictable, black swan events can have profound impacts on earnings. By incorporating extreme scenarios into our forecasts, we can better prepare for and respond to these high-impact events.
Using asymmetric bets: identifying scenarios with disproportionate upside or downside potential can uncover good investment opportunities or highlight significant risks that might otherwise be overlooked.
Mitigating psychological biases: awareness of our cognitive biases is the first step in mitigating their impact. By implementing structured processes and leveraging diverse perspectives, we can work to overcome these inherent mental shortcuts and improve the objectivity of our forecasts.
As we conclude, I want to emphasize that scenario forecasting is not just a technical exercise—it's a mindset. It's about embracing uncertainty, thinking critically about the future, and preparing for a range of potential outcomes.
Further Reading
Books
"Thinking, Fast and Slow" by Daniel Kahneman
Explores cognitive biases and their impact on decision-making.
"The Black Swan: The Impact of the Highly Improbable" by Nassim Nicholas Taleb
Discusses the role of rare, high-impact events in various domains, including finance.
"Superforecasting: The Art and Science of Prediction" by Philip E. Tetlock and Dan Gardner
Examines the characteristics of successful forecasters and their methods.
"Expectations Investing" by Michael J. Mauboussin and Alfred Rappaport
Provides insights into using expectations analysis for investment decisions.
"The Misbehavior of Markets: A Fractal View of Financial Turbulence" by Benoit Mandelbrot and Richard L. Hudson
Challenges traditional financial theories and offers new perspectives on market behavior.
Articles and Research Papers
"Base Rates: A Practical Guide" by Michael J. Mauboussin
Available on the Morgan Stanley website.
"Scenario Planning: A Tool for Strategic Thinking" by Paul J.H. Schoemaker
Published in Sloan Management Review.
"Decisions Under Uncertainty: Heuristics and Biases" by Amos Tversky and Daniel Kahneman
Classic paper on cognitive biases, published in Science.
"The Earnings Forecast Accuracy of Financial Analysts" by Mark T. Bradshaw et al.
Published in the Journal of Accounting Research.
“How to Improve Forecasting Ability Using Findings in Meteorological Science” by Polymath Investor
Online Resources and Courses
Coursera: "Model Thinking" by Scott E. Page
Covers various modeling techniques applicable to scenario forecasting.
edX: "Analyzing and Visualizing Data with Excel" by Microsoft
Useful for developing quantitative skills for scenario analysis.
Nafrada, C., & Caddell, J. (2021, December 7). ‘Never Thought They Could Pull Off Such an Attack’: Prejudice and Pearl Harbor. War on the Rocks. https://warontherocks.com/2021/12/never-thought-they-could-pull-off-such-an-attack-prejudice-and-pearl-harbor/
Svenson, O. (1981). Are we all less risky and more skillful than our fellow drivers? Acta Psychologica, 47(2), 143-148