When Everyone Codes at Warp Speed... Where’s the Moat?
A look into the AI coding revolution: What every investor has to know before investing in software companies and how Cursor and Windsurf are sending shockwaves to the industry
Picture this: it’s 10 p.m. on a Thursday.
Let’s say Alex, a mid‑level developer at a sleepy SaaS company, opens his laptop with a wild idea—build a fully‑functional subscription billing feature before dawn. Twelve hours later, coffee‑stained but grinning, he hits “deploy.” The codebase is clean, the tests pass, and customers are already signing up.
Alex didn’t grow an extra pair of hands overnight; he hired an invisible army. Its name? Cursor.
Swap Cursor for Windsurf and the story plays out the same. In less than two years, that first coding tool became the fastest-growing SaaS product of all time, skyrocketing from $1 million to $100 million in annual recurring revenue in just 12 month.1
For us investors, such meteoric growth and adoption are clear signals: a fundamental shift in software development is underway. Something deep inside software’s economic engine just shifted a gear.
So, which are the implications for investing in software companies?
How are competitive advantages going to be re-shaped?
Who are the winners and losers under this new paradigm?
In today’s article I’ll try to break down that shift and explore how AI coding assistants are sending shockwaves through the software industry.
Meet Cursor and Windsurf: Why This Isn’t Just “Better Autocomplete”
Cursor and Windsurf are the two leading examples of a new class of AI-native coding tools that are transforming how software is written.
So, what do they do?
In essence, they function like AI pair programmers embedded in the development environment, using large language models trained on billions of lines of code to assist many aspects of coding.
What’s the difference to a usual coder workflow?
Well, as I understand it, traditionally, a developer used to write code manually, one line at a time, using an editor or IDE (Integrated Development Environment). Now, with Cursor or Windsurf, he/she can describe an intent or ask a question in natural language, and the tool will generate code, suggest improvements, or even execute commands across an entire project.
This last part is key, the tool won’t just suggest a line of code in a specific file but it will generate changes in files across the project to deliver on the feature being asked by the developer.
What’s remarkable is how quickly these tools have matured and been adopted. By late 2024 Cursor reached around 360,000 paying users and about $100 million in ARR, a growth trajectory faster than any SaaS company before it.
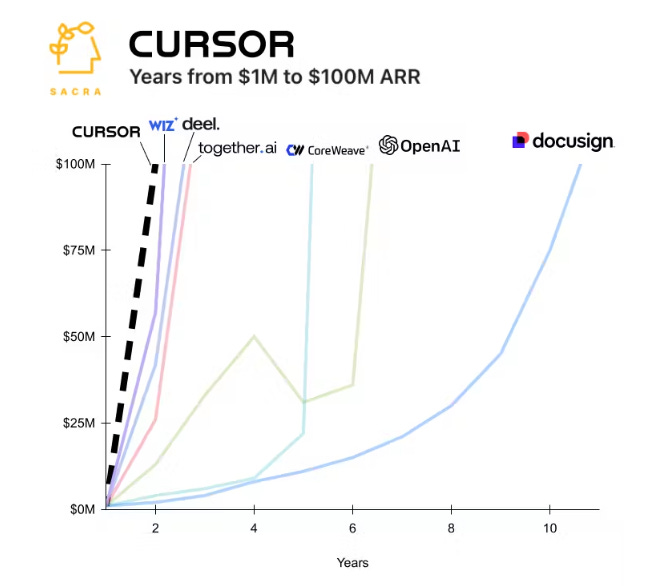
A Fundamental Shift in How Software Is Built
Software development has historically been constrained by human developer productivity.
Writing good code is, or was, an artisanal process: a skilled but expensive and time-consuming craft.
Over decades, higher-level programming languages were created, reusable libraries developed, and tools like code editors and debuggers became popular– all aimed at making developers more efficient.
So what’s different now? In the past, even with all the code editors and debuggers, creating complex software still required large teams and many months. Now, AI coding offers an order-of-magnitude improvement.
Here is where it gets relevant for investors.
AI-assisted coding changes the unit economics of software creation.
If a single developer can accomplish in a day what might have taken a team a week, the implications are well…massive. And this is just today’s AI; the models are improving rapidly.
Beyond raw speed, AI coding tools also alter the nature of the development process.
Development can become more iterative and exploratory, because the cost (in time and effort) of trying an idea is so much lower.
The human developer becomes a curator and coach, focusing on whether the software behaves as intended, rather than on typing out every semicolon.
According to some forecasts2 90% of code may eventually be AI-generated, but does this means that engineers will be obsolete? I don’t know, but I think that the Jevons Paradox3 may apply here.
When the cost of coding plummets, imagine the implication that that has for capital allocation decisions. How many more projects will become viable?
The demands for capable engineers will probably explode.
Why?
Because when each engineer’s output is amplified by AI, the return on investing in software development skyrockets.
Companies will take on projects that were previously impractical due to cost or time. And if software was eating the world before… in this new paradigm, as it becomes ubiquitous, software will devour every last corner of it.
The question every tech company will have to ask is “How fast can we deploy new technology before our competitors do?”
Expect roadmaps to double in breath, not halve in headcount.
Consequences for Productivity, Costs, and Development Cycles
So, which are the implications at a company level? Which are the metrics we, as investors, should pay attention to?
Let’s review a few in the table below.
So the promise of the AI coding revolution guarantees cheaper, faster, and potentially better software development.
But wait, what does this mean for competitive advantages in the software industry?
If everyone has access to these AI tools, do any advantages still remain? Are these tools the Trojan Horse that could finally dismantle Big Tech’s moats?
Lets see.
Where’s The Moat Now?
Sustainable competitive advantages or moats in the software world have traditionally come from switching costs, data, network effects, and, if you want to use a loose definition of moat, you can include superior talent, sophisticated code or the ability to innovate faster than others.
Now whats’s going to happen when everyone can code at warp speed?
Let’s see.
1. Lower Barriers to Entry (and Faster Imitation)
One immediate effect is that it becomes easier for a small team – or even an individual – to build a complex application. This lowers barriers to entry in many software markets. It means that any feature-based moat (where a company’s advantage is that it has more features or better implementation) becomes smaller. In the future, the time lag for competitors to catch up will shrink. Companies will have to rely on continuous innovation and customer lock-in (think supercharged switching costs, propriertary data or network effects). I’m thinking out loud here, but what are the implications for serial software acquirers like Constellation Software ($CSU.TO), if hundreds of mission-critical vertical software companies could be replicated—or even replaced—in a matter of weeks?
2. The Importance of Unique Data and Proprietary Context
If everyone has access to similar AI models and coding knowledge becomes commoditized, where can a durable moat come from? One answer, as I mentioned above, is proprietary data. Companies that own unique datasets can fine-tune or guide AI models in ways others can’t. Thus, data network effects (where more users = more data = better AI = more users) could become a key competitive flywheel.
3. Talent and “AI-Native” Cultures
Great engineers have always been a competitive advantage. Now the definition of “great” might shift towards those who can best leverage these AI tools effectively. Think about it, you still have to know how to code but the skills required to be successful may change a little bit. Also, does the company culture foster the use of these new tools or the old way of doing things prevail? There’s an innovation Tsunami coming, and many organizations could go underwater.
4. Shifting Value to Design and Strategy
So, if implementation (the act of writing code) becomes faster and easier, the relatively scarcest skills shift upstream to product design, vision, and strategic insight. It will be more important to come up with the right thing to build rather than just building something. You will have to have an intimate understanding of customer needs because getting an idea coded is no longer the bottleneck. This favours businesses with strong user communities or feedback loops (so they know what to build) and with leadership that encourages experimentation (since the cost of failure is lower now).
5. High Switching Costs Still Stands
Moats like ecosystem lock-in or integration depth might still hold. Large enterprise software often wins because it integrates deeply into a company’s processes – AI can help newcomers build features, but integration and trust take time. Brand and customer trust will also remain important; an AI-generated clone of Salesforce doesn’t automatically get Salesforce’s customers.
Sector-by-Sector Impact: Which Software Companies Will Be Most Affected?
Every sector is going to be affected in a different way. As I mentioned above, some are more protected than others.
Take, for example, a SaaS company that develops software for banks or asset managers. These relationships take time to build, and trust and security are paramount. So, I don’t expect much disruption there—at least not initially.
But what happens when the broader market narrative around software shifts? Will multiples compress across the board? Will the baby be thrown out with the bathwater?
Time will tell. If that happens, there will be opportunities for those who can separate the wheat from the chaff.
In the table below, I’ve included some pros and cons by sector:
Conclusion
There’s a profound transformation coming, and I wanted to put this article out also to clear my thoughts on this matter.
Here are a few things I will add to my software companies checklist that I think could be helpful in assessing the exposure to this new environment:
Understand AI‑Readiness
Are the engineering teams deploying AI copilots, automated testing, and code‑gen tools?
Companies embracing these platforms can release features faster and cheaper.
Re‑interpret Productivity Ratios
We will probably see increases in revenue or new feature releasing jumps without a proportional increase in R&D, start thinking on AI leverage.
On the same topic, don’t penalize companies reinvesting AI‑driven savings into new products—offensive deployment of AI “dividends” can expand total addressable market.
Stress‑Test Competitive Moats Under 10× Cheaper Code
Simple, first‑mover products are easier to clone when AI cuts build costs; brands with proprietary data, strong communities, or regulatory licenses remain sturdier.
Favour businesses that feed unique data back into their models, compounding an AI‑powered network effect.
Value Human Capital
Expect more acqui‑hires focused on securing cohesive, high‑AI‑fluency teams rather than just codebases.
Plan for Rapid Product Cycles and Higher Volatility
Differentiating features today may become commodity within a year as AI accelerates imitation and innovation.
Cursor and Windsurf’s explosive rise is a harbinger of things to come – they demonstrate what’s possible when AI is integrated into the fabric of coding.
For those of us evaluating companies, it’s time to ask anew: In the age of AI-assisted coding, what truly makes this business defensible and exceptional? The answers will guide smarter investment decisions in the transformative years ahead.
Disclaimer
This newsletter (the “Publication”) is provided solely for informational and educational purposes and does not constitute an offer, solicitation, or recommendation to buy, sell, or hold any security or other financial instrument, nor should it be interpreted as legal, tax, accounting, or investment advice. Readers should perform their own independent research and consult with qualified professionals before making any financial decisions. The information herein is derived from sources believed to be reliable but is not guaranteed to be accurate, complete, or current, and it may be subject to change without notice. Any forward-looking statements or projections are inherently uncertain and may differ materially from actual results due to various risks and uncertainties. Investing involves significant risk, including the potential loss of principal, and past performance is not indicative of future results. The author(s) may or may not hold positions in the securities discussed. Neither the author(s) nor the publisher, affiliates, directors, officers, employees, or agents shall be liable for any direct, indirect, incidental, consequential, or punitive damages arising from the use of, or reliance on, this Publication.
Asplund, J. E. (2025, February 5). Cursor at $100M ARR. Sacra. https://sacra.com/reports/cursor-100m-arr
Rachitsky, L. (2025, April 20). Building a magical AI code editor used by over 1 million developers in four months: The untold story of Windsurf | Varun Mohan (co-founder & CEO). Lenny's Newsletter. https://www.lennysnewsletter.com/p/building-magical-ai-code-editor
The Jevons Paradox refers to the counterintuitive idea that increasing the efficiency with which a resource is used tends to increase, rather than decrease, the overall consumption of that resource. It was first observed by economist William Stanley Jevons in the 19th century, who noticed that improvements in coal-use efficiency led to more coal consumption, not less—because cheaper usage made coal more economically attractive, boosting demand. In short: more efficiency → lower cost → higher demand → more total use
Great analysis. Your take on it makes me realize that the future in AI is unknowable. The advances are so rapid, it is impossible to know who wins. As a value investor, I am going to shift my focus of reasearch elsewhere, while everyone looks at AI in amazement. Thank you for your content.